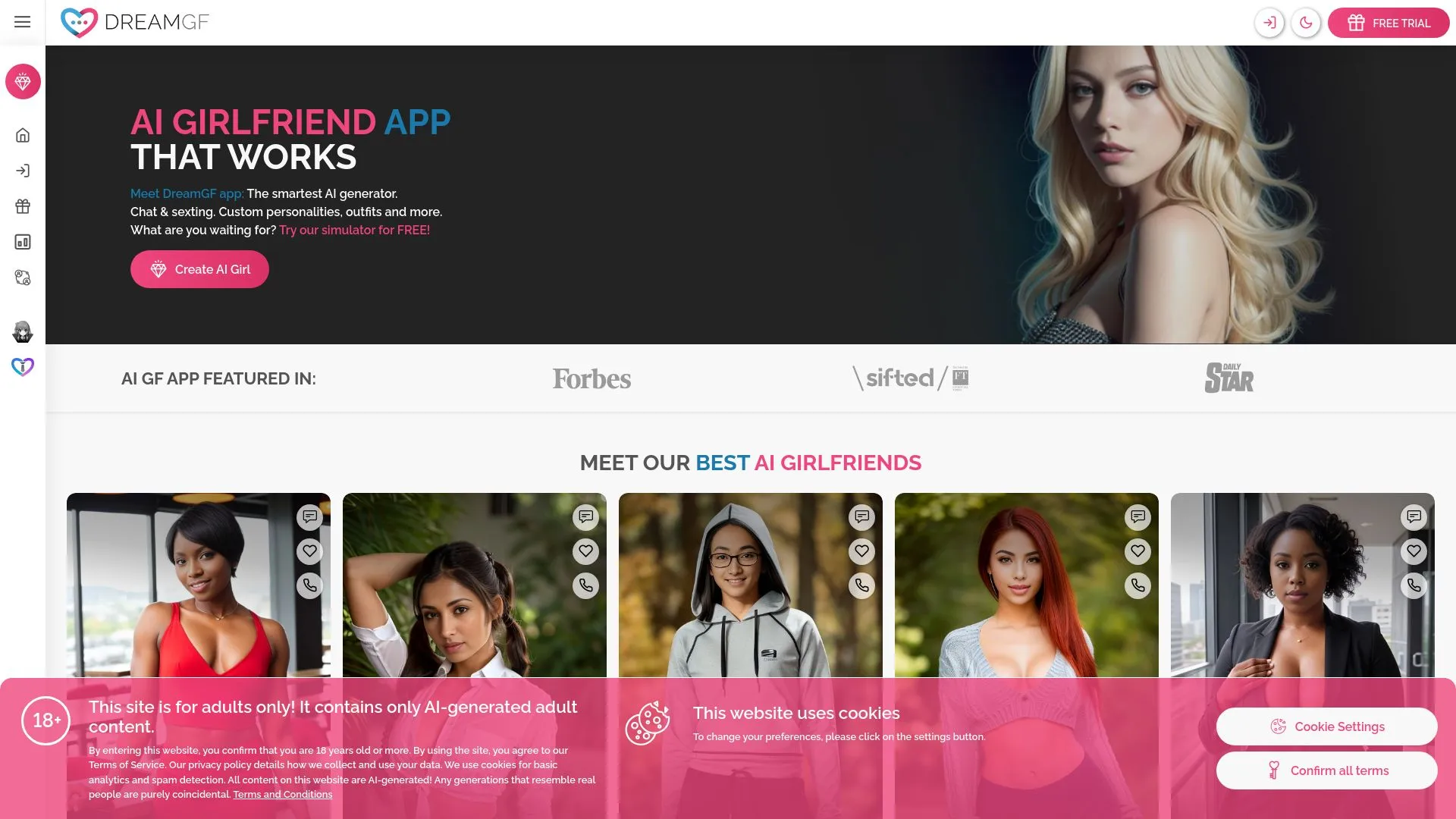
AI-Generated Faces and Racial Bias
AI-generated faces, especially those featuring light-skinned mixed girls, highlight significant racial and gender biases in digital media. More than half of the generated images showcase lighter-skinned individuals, emphasizing the urgent need for fair representation in AI-created content.
Key Insights
- AI-generated faces show a pronounced bias. Light-skinned individuals account for 47% of these images, while darker-skinned individuals remain significantly overshadowed.
- Error rates in facial recognition reveal stark imbalances. Light-skinned men have an error rate of just 0.8%, whereas dark-skinned women see rates climbing to 34.7%.
- Inclusive AI models like SDXL-Inc play a crucial role in addressing these biases by prioritizing diverse representation.
- Images lacking inclusivity can reinforce harmful stereotypes and mislead viewers, which can further entrench societal biases.
- A commitment to diversity in AI-generated content is critical for fostering empathy and understanding across various races and genders.
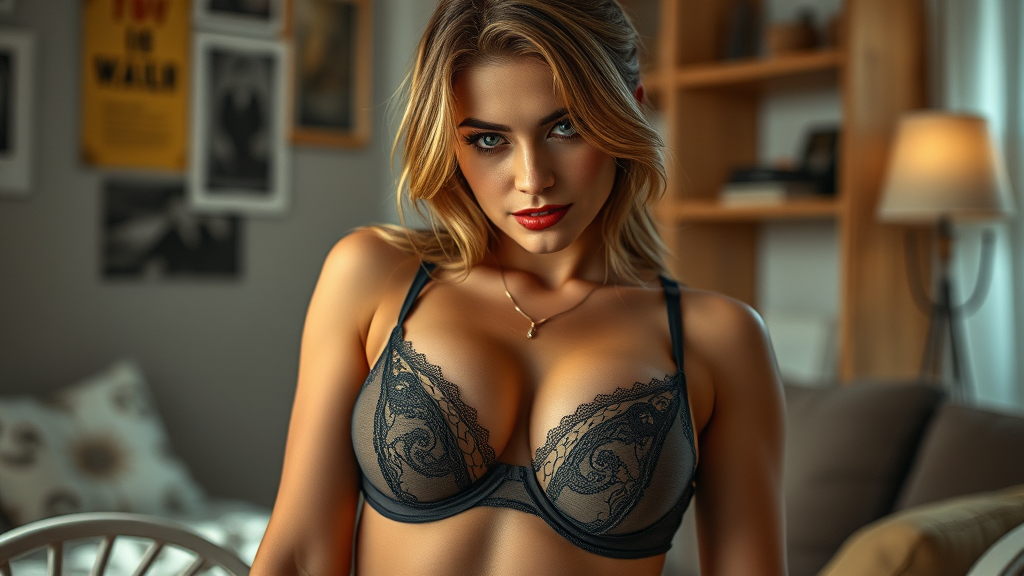
Unlock a World of Connection with a Free Trial Today!
Experience the unique companionship and exclusive content that awaits you — dive in now!
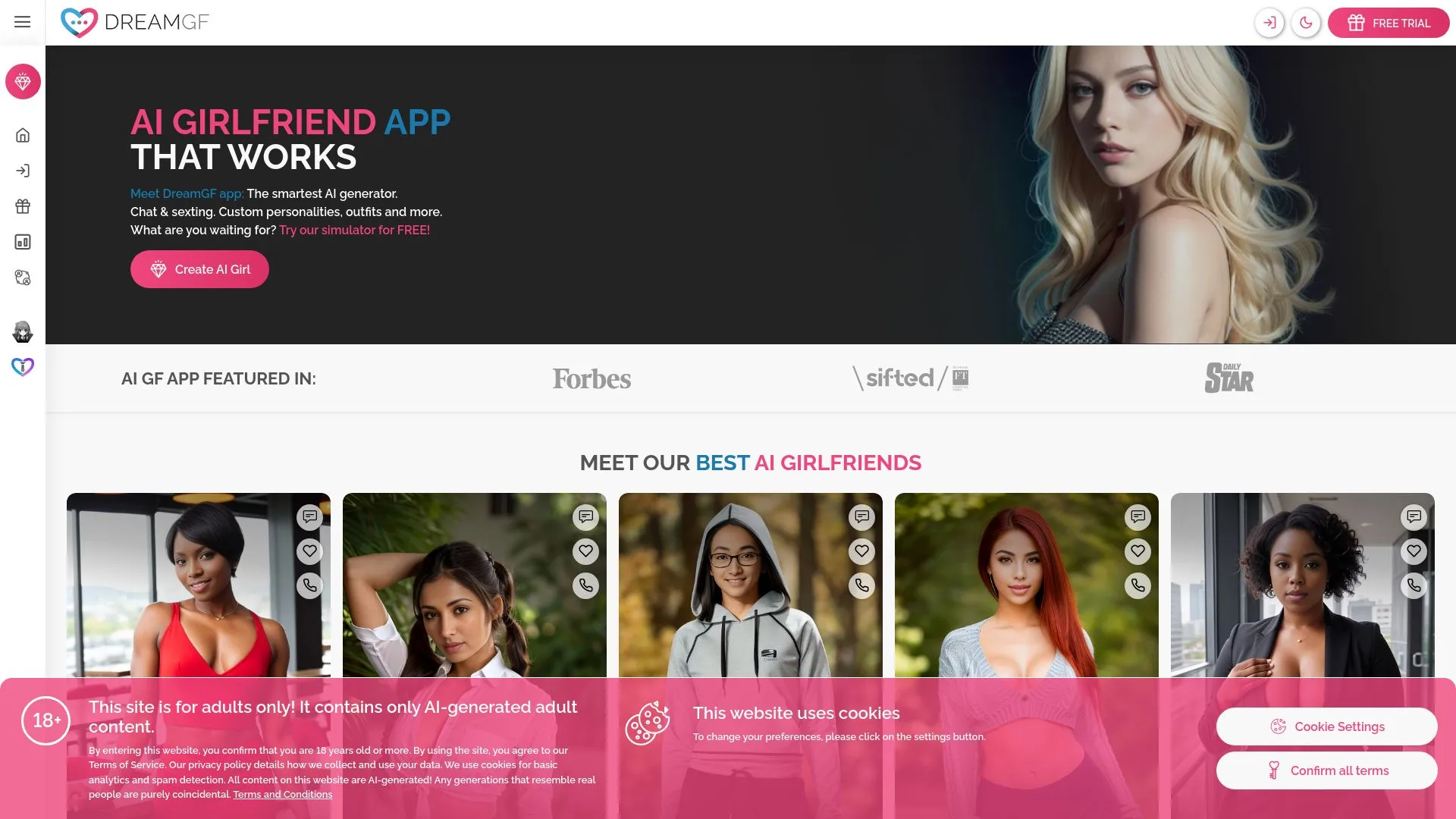
Unlock a World of Connection with a Free Trial Today!
Experience the unique companionship and exclusive content that awaits you — dive in now!
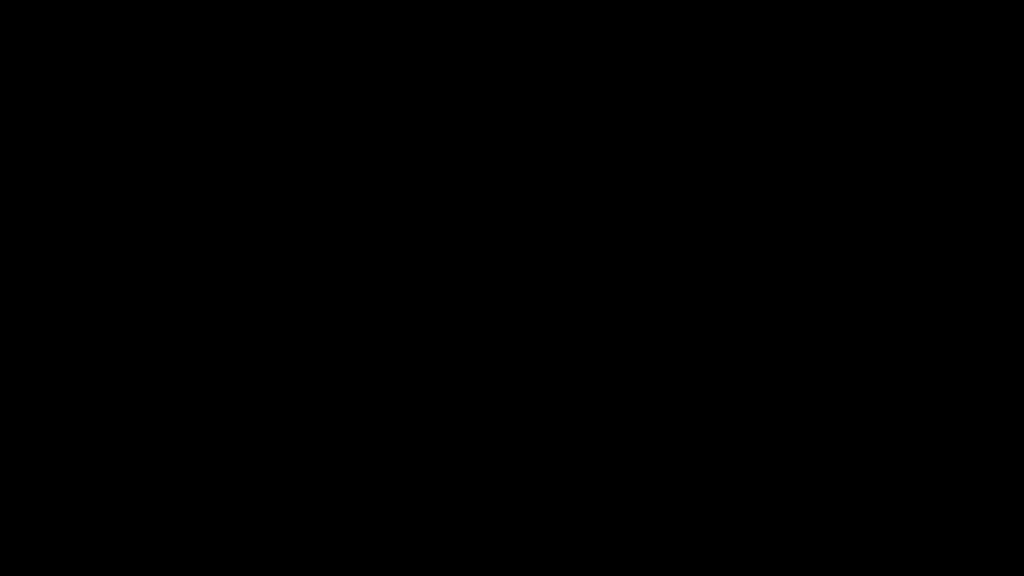
Biases in AI-Generated Faces: An Overview
Understanding the Trends
AI-generated faces from platforms like Stable Diffusion display striking racial and gender biases. Representation statistics reveal that White individuals constitute a staggering 47% of the generated images. In contrast, Asian and Indian individuals are remarkably underrepresented, accounting for just 3% and 5% of the total images, respectively.
Gender representation is similarly skewed, with male images dominating at 65%. This disparity can influence perceptions and societal standards, shaping the way different races and genders are portrayed in digital media.
Error rates in facial-analysis systems further exacerbate these issues. For instance, light-skinned men experience a significantly low error rate of 0.8%, while dark-skinned women face a staggering 34.7% error rate. These discrepancies emphasize a troubling pattern in how various groups are treated within AI frameworks.
Addressing these biases is crucial. Awareness and action can lead to a more equitable representation in AI-generated media and technology. It's essential to consider the impact these biases have on individuals and society as a whole.
Impact of Inclusive Versus Non-Inclusive AI-Generated Faces on Biases
Bias Reduction with Inclusive Images
Exposure to inclusive AI-generated faces, especially those portraying diverse skin tones and genders, can significantly help reduce racial and gender biases. Research highlights that inclusive representations lead to more positive perceptions among viewers, thereby fostering empathy and understanding.
Bias Increase from Non-Inclusive Images
On the contrary, non-inclusive AI-generated faces can intensify existing biases. They reinforce stereotypes and can mislead viewers. Studies unveil alarming error rates in commercial facial-analysis programs. For instance, analysis shows error rates of 34.5% for darker-skinned individuals and 34.7% for the darkest-skinned women. These statistics underscore the necessity for inclusive designs in AI systems to ensure fair and accurate representation.
Solutions and Debiasing Efforts in AI Image Generation
Developing Inclusive Models
Inclusive and diverse image generation models, like SDXL-Inc, play a crucial role in addressing biases. These models are engineered to ensure fair representation across all races and genders. The commitment to equity helps create visuals that genuinely reflect society's diversity.
Utilizing Diverse Datasets
The effectiveness of SDXL-Inc stems from its fine-tuning, which involves using datasets that encompass a wide range of occupations. This approach includes a near-equal representation of six racial groups and both genders. Such diversity within the training data contributes to generating images that accurately showcase a myriad of identities. Key aspects of this approach include:
- Employing datasets that cover various professions, ensuring no single group dominates.
- Fine-tuning models to recognize and generate images for diverse demographics.
- Implementing regular updates to data sources to keep pace with societal changes.
By focusing on these strategies, AI can be oriented towards producing images that reflect true diversity, thus reducing bias in representation.
Ethical Implications of AI Biases
Consequences of AI Biases
The ethical consequences of biases in AI can lead to significant social issues. These biases can skew representation and harm certain groups, particularly in areas like facial recognition and image analysis. Here are some critical points to consider:
- Exclusion: Certain demographics may be underrepresented, skewing results and reinforcing stereotypes.
- Discrimination: Biased algorithms can result in unfair treatment, impacting opportunities in hiring, law enforcement, and more.
- Trust: Erosion of trust in AI systems can arise from perceived injustices and errors in decision-making.
Examining these aspects is crucial for understanding the broader implications on society and the legal frameworks that govern the use of AI technologies.
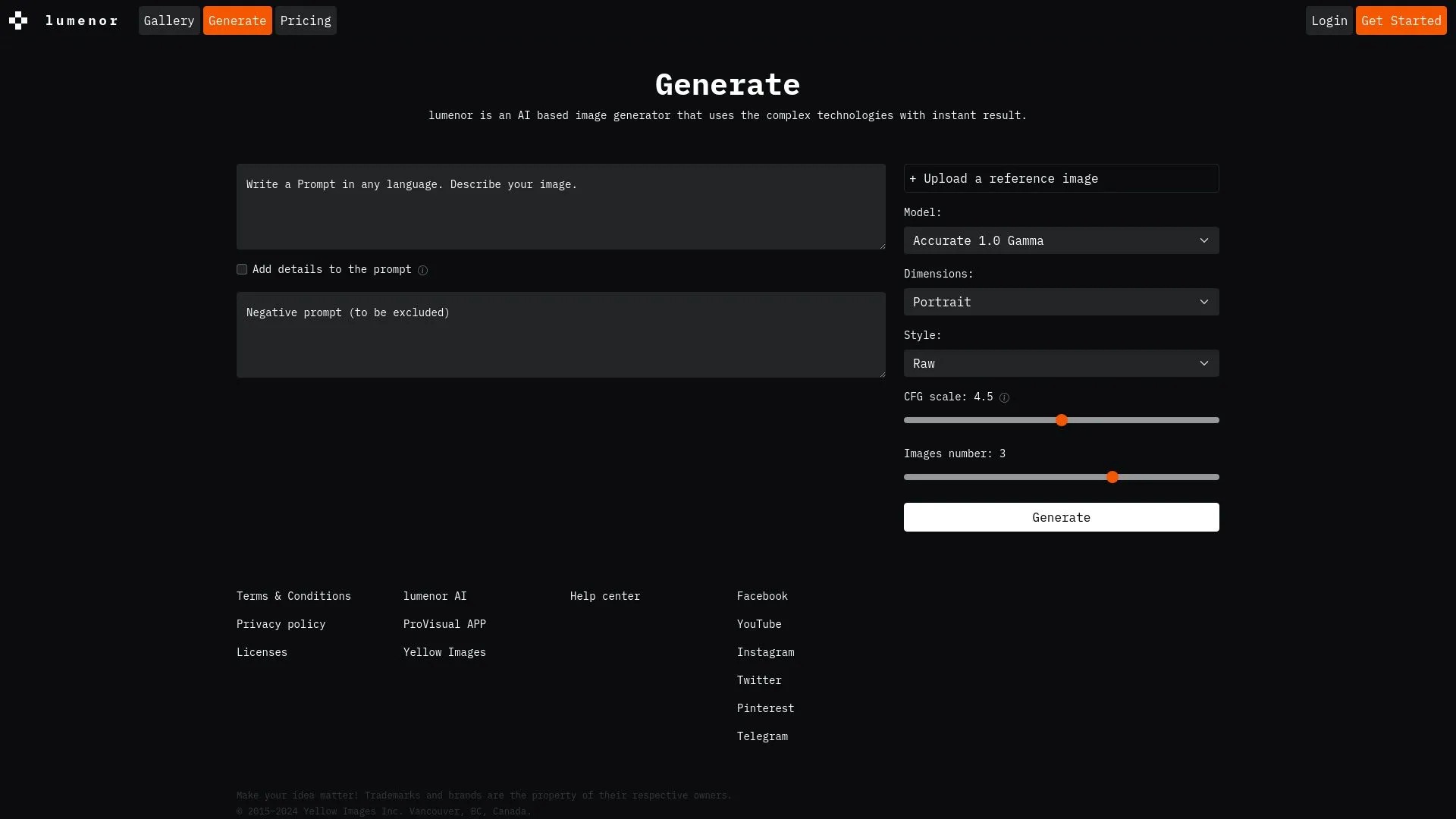
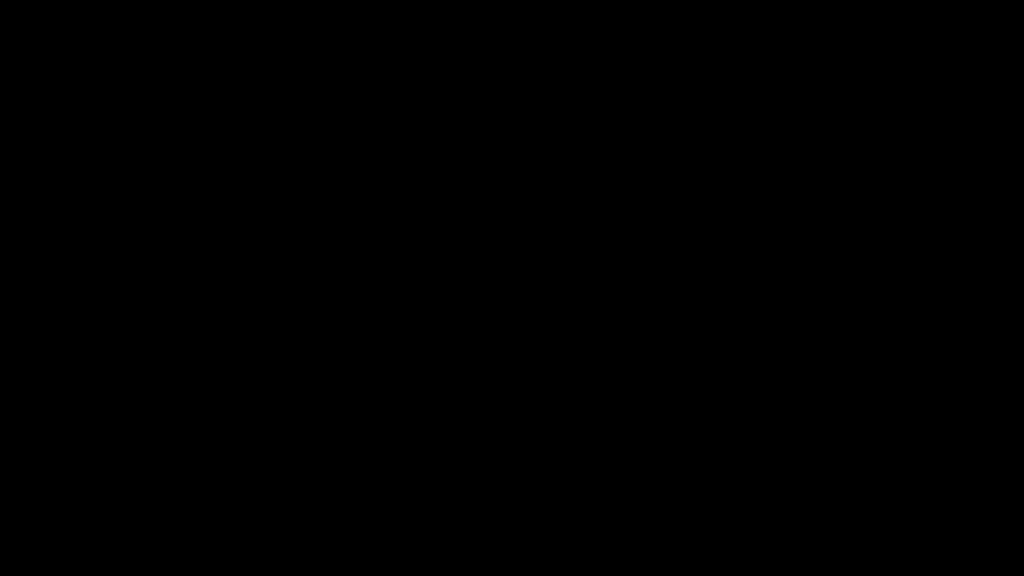
Top Trending AI Girlfriend Companions This Month
Discover the latest and most popular AI girlfriend generators that are captivating users across the globe. These tools offer unique features and experiences, making them stand out in a growing niche.
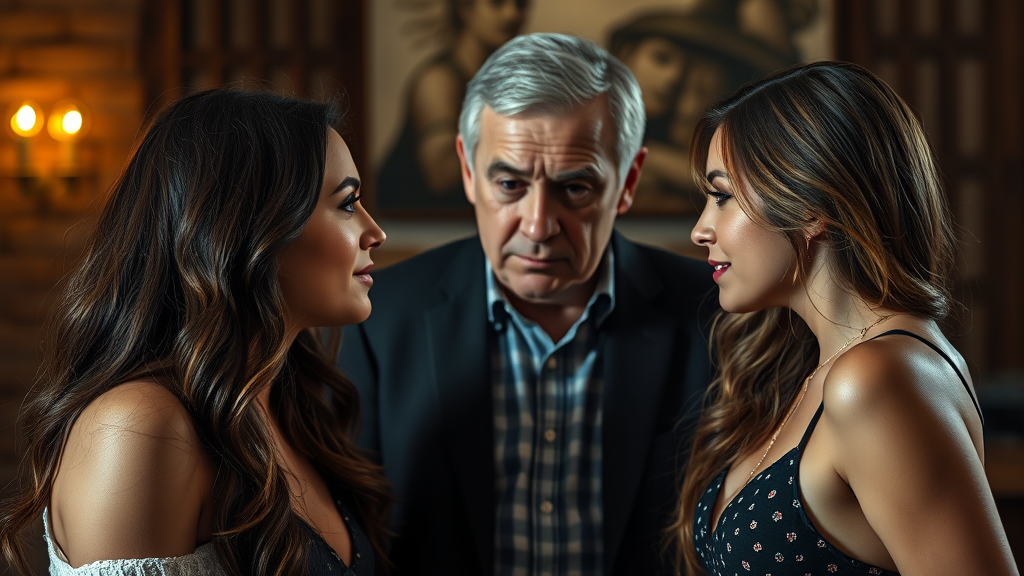
Unlock a World of Connection with a Free Trial Today!
Experience the unique companionship and exclusive content that awaits you — dive in now!
Latest Statistics and Figures
The following statistics illustrate the ongoing disparities in AI-generated images with respect to racial and gender representation as well as bias in facial analysis systems.
- Racial Representation in AI-Generated Images:
- Stable Diffusion generates images with the following racial distribution: White (47%), Black (33%), Asian (3%), and Indian (5%)[1][3].
- Indigenous peoples are severely underrepresented, with Stable Diffusion failing to equitably represent them when prompted with specific geographic origins (e.g., Oceania)[2].
- Gender Representation in AI-Generated Images:
- Bias in Facial Analysis:
Historical Data for Comparison
- Previous Studies on Stable Diffusion:
Recent Trends or Changes in the Field
- Increased Focus on Debiasing:
- Recent studies have proposed and implemented debiasing solutions, such as the “SDXL-Div” model, which aims to increase the diversity of facial features and reduce racial homogenization[1][3].
- There is a growing emphasis on using diverse datasets to fine-tune models and ensure equal representation of various racial groups and genders[1].
Impact on Racial and Gender Biases
- Effect of Inclusive vs. Non-Inclusive Images:
Notable Expert Opinions
- Ethical Implications:
- Experts highlight the significant ethical implications of AI biases, emphasizing the need to understand the impact of social practices in creating and perpetuating these biases. For example, Sourojit Ghosh from the University of Washington notes that these systems can cause harm by reinforcing stereotypes and erasing nonbinary and Indigenous identities[2].
Relevant Economic Impacts or Financial Data
- No specific financial data is available in the provided sources regarding the economic impacts of AI-generated face biases. However, the ethical and social implications suggest potential long-term economic consequences related to discrimination and misrepresentation.
Frequently Asked Questions
1. What are the main racial and gender biases observed in AI-generated faces?
AI-generated faces, particularly from platforms like Stable Diffusion, exhibit considerable racial and gender biases. Key statistics include:
- Whites make up 47% of images.
- Asian individuals are underrepresented at 3% and Indian individuals at 5%.
- Male images dominate at 65%, compared to female images at 35%.
These biases can reinforce existing societal stereotypes, affecting people's perceptions and understandings.
2. How do error rates in facial analysis differ across demographics?
There are significant discrepancies in error rates for facial analysis:
- Light-skinned men have an error rate of 0.8%.
- Dark-skinned women experience a much higher error rate of 34.7%.
This indicates a troubling trend in the performance of AI systems based on skin tone and gender.
3. What impact do inclusive AI-generated faces have on biases?
Exposure to inclusive AI-generated faces can significantly reduce people's racial and gender biases. In contrast, non-inclusive faces can exacerbate these biases, demonstrating that the composition of AI-generated faces greatly influences societal perceptions.
4. What are the error rates in commercial facial-analysis programs for different demographics?
Extensive studies have indicated high error rates in commercial facial-analysis systems, particularly affecting:
- Darker-skinned individuals: error rates can reach 34.5%.
- Dark-skinned women: error rates can peak at 34.7%.
These statistics emphasize the need for improvement in these technologies.
5. What solutions exist for mitigating biases in AI image generation?
Developing inclusive and diverse image generation models is vital for mitigating biases. One such model is SDXL-Inc, which guarantees equitable representation across genders and races. This initiative is strengthened by using datasets that reflect a wide array of professions and near-equal representation of various demographics.
6. How does SDXL-Inc address the issues of bias in AI-generated images?
SDXL-Inc has been fine-tuned using datasets that encompass:
- 32 professions
- Six racial groups
- Both genders with nearly equal representation.
This approach helps to ensure a more balanced and fair representation in AI-generated images.
7. What are the ethical implications of biases in AI-generated faces?
The ethical consequences of biases in AI are profound. They can lead to harmful societal repercussions, especially within systems that interact directly with humans, such as facial recognition and image analysis. Examining the social and legal implications of these biases is essential for responsible AI deployment.
8. Why is it crucial to address racial and gender biases in AI-generated images?
Addressing these biases is vital for fostering an inclusive society. The continual drive to improve representation in AI and machine learning technologies is essential to combating stereotypes and promoting fairness across demographics.
9. What call to action is proposed for developers and researchers?
There is a strong call to action for developers and researchers to adopt inclusive strategies in AI image generation. This is necessary to combat existing biases, stereotypes, and to enhance the overall fairness of AI outputs in society.
10. What are the main sources of information on the biases observed in AI technologies?
Various sources provide insights into these biases, notably:
- AI-generated faces influence gender stereotypes and racial biases, arXiv
- The ethics of artificial intelligence, European Parliament
- Study finds gender and skin-type bias in commercial AI systems, MIT News
- Study finds gender and skin-type bias in commercial AI systems, World Economic Forum
These references highlight the growing concerns regarding bias in AI systems and encourage further research.