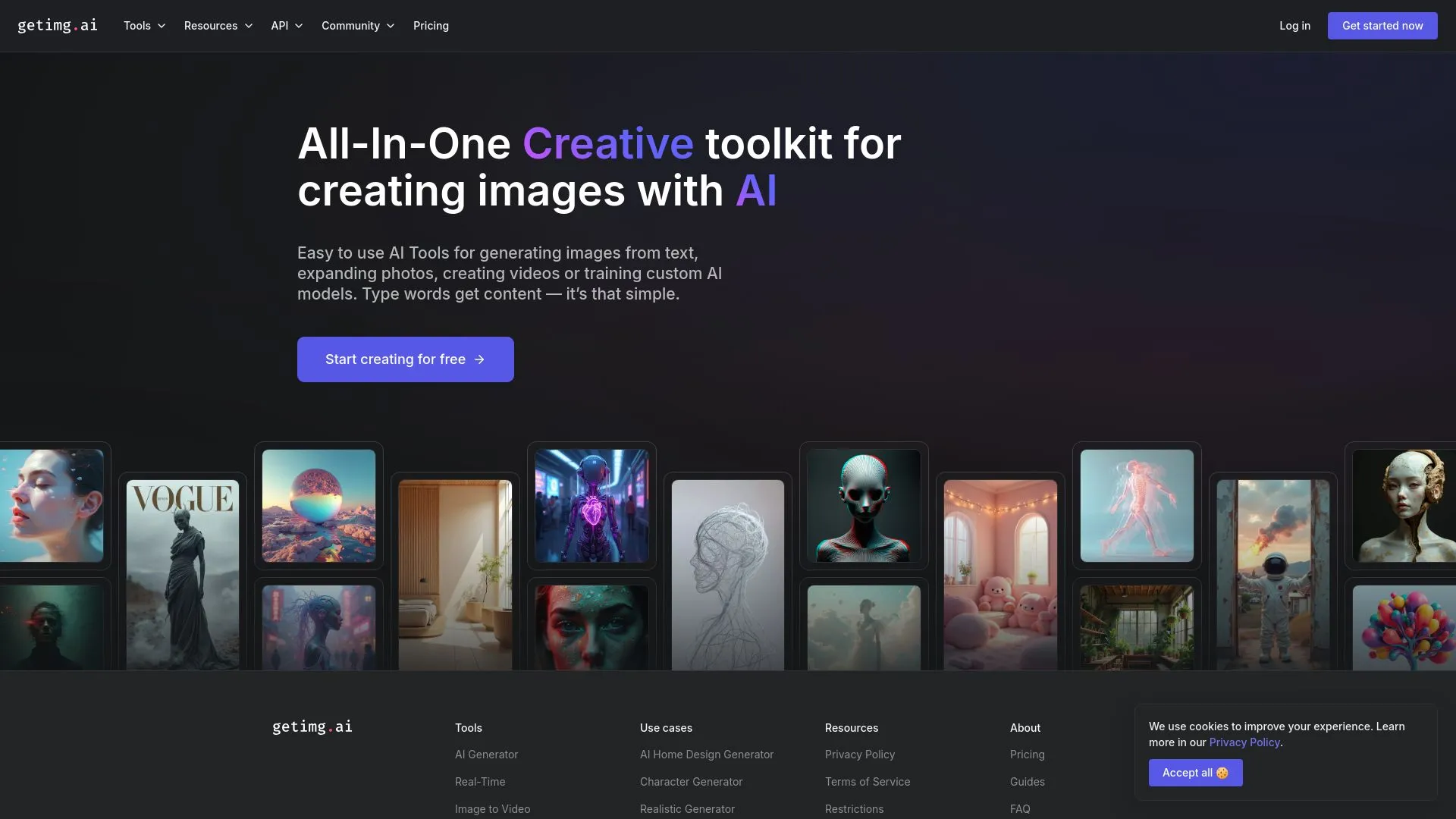
AI-generated images can inadvertently reinforce existing racial and gender biases. This leads to the significant underrepresentation of diverse groups, such as mixed-race individuals. Tackling these biases is crucial for creating fair imagery that truly represents societal diversity. There’s a clear need for more inclusive datasets and effective strategies to reduce bias.
Key Takeaways
- AI systems often exacerbate racial and gender biases due to unbalanced datasets.
- Mixed-race individuals and diverse couples frequently face misrepresentation or are overlooked in AI-generated images.
- Representation within AI datasets is significantly skewed, with a predominance of White individuals.
- More inclusive AI-generated images can help alleviate biases and foster empathy, while exclusive images tend to reinforce stereotypes.
- Implementing bias-reduction strategies and broadening datasets are essential steps to achieving fair AI outputs.
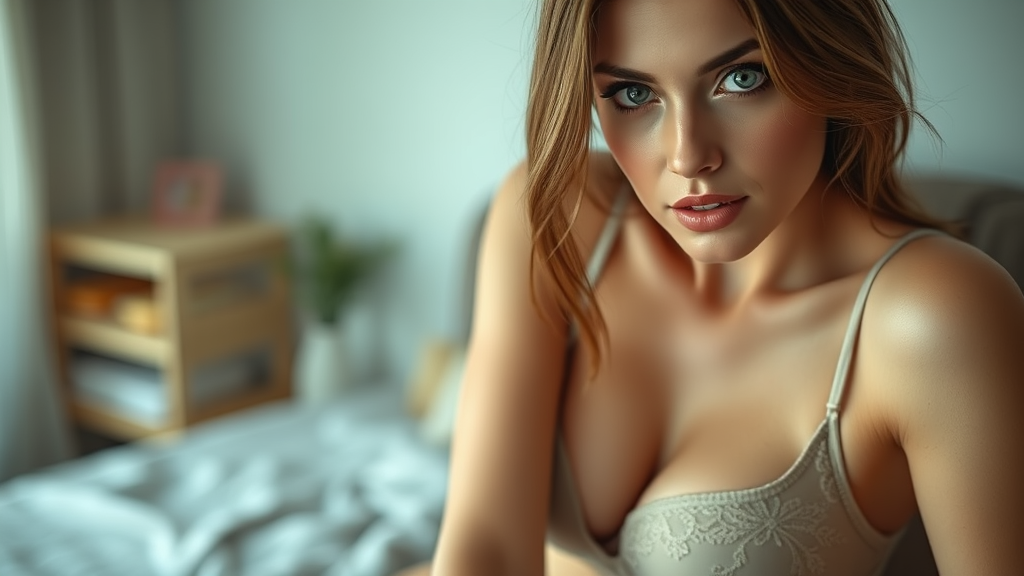
Unlock Exclusive Content with a Free Trial
Experience the unique connection and tailored content that awaits you with our trial offer.
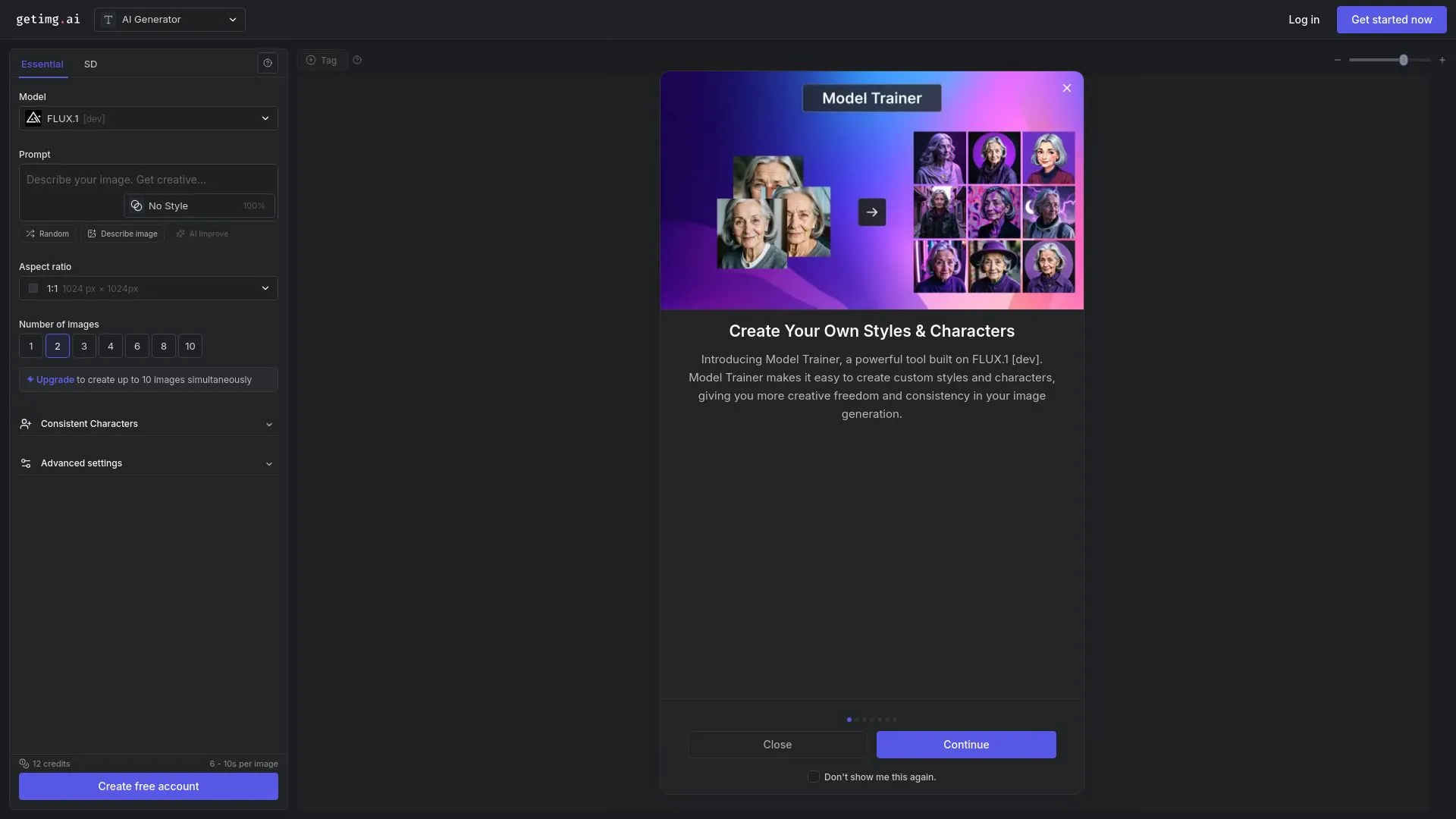
Unlock Exclusive Content with a Free Trial
Experience the unique connection and tailored content that awaits you with our trial offer.
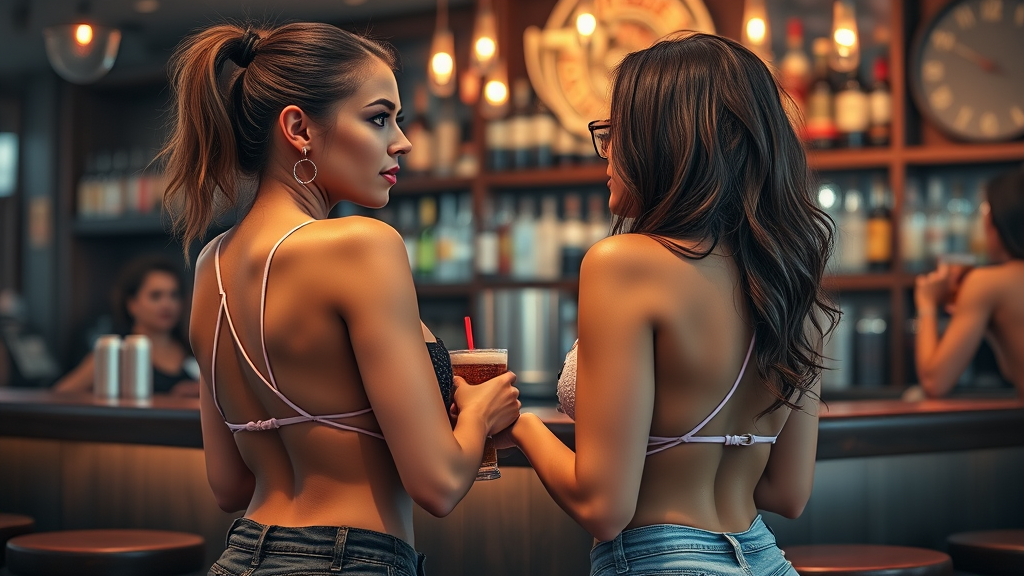
The Amplification of Racial and Gender Biases
AI-generated images frequently mirror and amplify prevailing societal biases, particularly regarding race and gender. A notable example is Stable Diffusion, which has been shown to misrepresent Middle Eastern men while exhibiting glaring discrepancies in face recognition accuracy. For instance, studies reveal that accuracy stands at around 99% for white males, but plummets to just 50% for Black women. This disparity illustrates how racial and gender biases are ingrained in AI systems.
The phenomenon of racial homogenization also contributes to these issues. When AI models generate faces, they may default to stereotypical features associated with dominant racial groups, further distorting representation. This reinforces existing norms and limits the diversity of faces produced, often sidelining mixed-race individuals entirely.
Such biases impact various sectors, from entertainment to security, where representation matters significantly. Recognizing these trends is crucial for anyone working with AI-generated content. Being aware of the limitations and shortcomings of these technologies helps in making informed choices that promote inclusivity and better representation. Engaging with diverse datasets and challenging the status quo is essential in creating more accurate and equitable AI systems.
The Underrepresentation of Diverse Races and Genders
AI-generated images highlight significant gaps in representation across diverse races and genders. Dataset disparities reveal that White individuals make up 47% of the dataset, while Black individuals account for 33%. Asians and Indians are severely underrepresented, comprising only 3% and 5%, respectively.
Gender representation shows a similar imbalance, with males dominating at 65% compared to just 35% for females.
These disparities lead to skewed AI outputs, contributing to stereotypes and limiting the visibility of diverse races. Ensuring a more inclusive approach in data collection and curation can enhance the authenticity and equity of AI-generated images. Steps include:
- Increasing diversity in datasets
- Actively seeking out underrepresented groups
- Regularly reviewing data for balance and inclusivity
Effects of Inclusive and Non-Inclusive AI Images
Impact of Inclusive AI-Generated Images
Exposure to inclusive AI-generated images can play a transformative role in reducing biases. These images help challenge existing racial and gender biases by providing Diverse representations. Increased exposure encourages viewers to broaden their understanding of different identities, which can foster empathy and acceptance.
Consequences of Non-Inclusive AI Images
On the contrary, non-inclusive AI images tend to amplify biases, regardless of being labeled as AI-generated. This limitation can reinforce stereotypes and narrow perspectives. It’s crucial to acknowledge the effects of exposure. Diverse visuals can cultivate an environment that promotes equality, while a lack thereof can perpetuate harmful biases. Prioritizing inclusive AI-generated images is essential for a more equitable society.
Challenges in Generating Diverse Images
Generating diverse images comes with significant hurdles, especially concerning mixed-race couples. A common issue arises in the representation of these relationships, where AI tools, like those by Meta, struggle to accurately depict Asian and white pairings. Often, the output leans heavily into clichés, failing to reflect the complexity and beauty of such unions.
This leads to a couple of notable challenges:
Key Challenges in AI Image Generation
- Diversity Challenges: Many AI systems don’t have comprehensive datasets that include a wide variety of mixed-race relationships. This lack of representation causes biases in the generated imagery.
- Stereotypes in AI Images: A recurring problem is that when AI tries to create images involving Asian women, it often falls back on stereotypes. Rather than capturing genuine diversity, it produces visuals that reinforce outdated notions.
AI image generation needs to evolve beyond these limitations. Fostering a richer dataset could lead to better representation, striking a balance between accuracy and creativity. Understanding these challenges is crucial for users aiming to create truly diverse imagery.
Advancements Through Debiasing Solutions
The Impact of Diverse Datasets
Research initiatives like the SDXL-Inc model highlight the importance of fine-tuning with diverse datasets. This approach significantly boosts dataset representation, aiming for nearly equal visibility of all races and genders. Traditional models like Stable Diffusion lacked this level of balance, showcasing disparities in representation. The SDXL-Inc model illustrates how incorporating varied data leads to improved model performance. By emphasizing fairness, these advancements help create a more inclusive AI landscape.
Critical Debiasing Solutions
Implementing debiasing solutions involves several key strategies:
- Data augmentation: Expanding datasets through the inclusion of underrepresented groups.
- Algorithmic adjustments: Tweaking model parameters to mitigate bias during training.
- Evaluation metrics: Establishing robust benchmarks to assess fairness and accuracy across demographics.
These methods contribute to refining AI outputs, ensuring that lighter mixed-girl representations come across more genuinely and equitably. This shift isn’t just beneficial; it’s essential for cultivating a trustworthy AI environment.
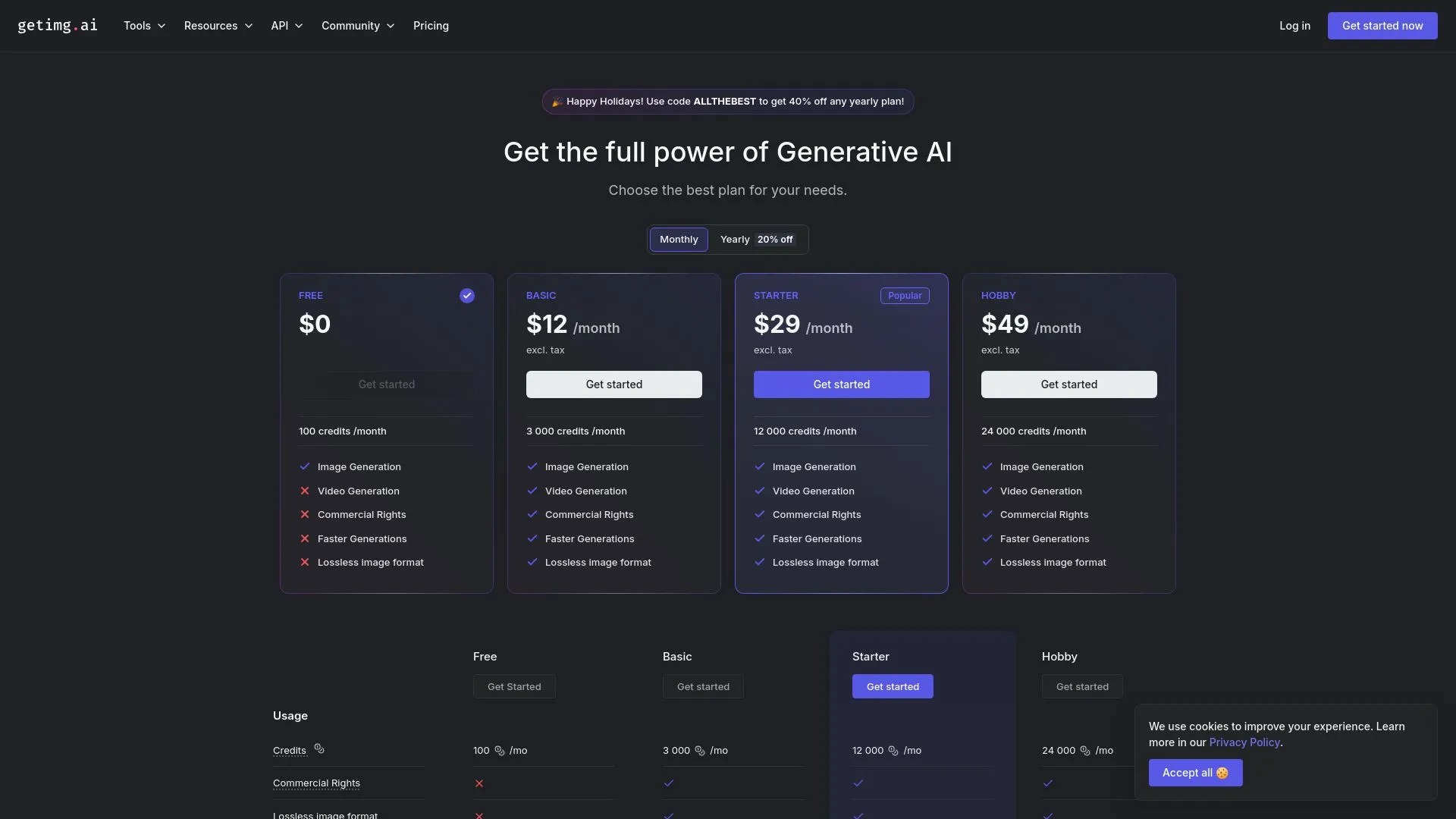
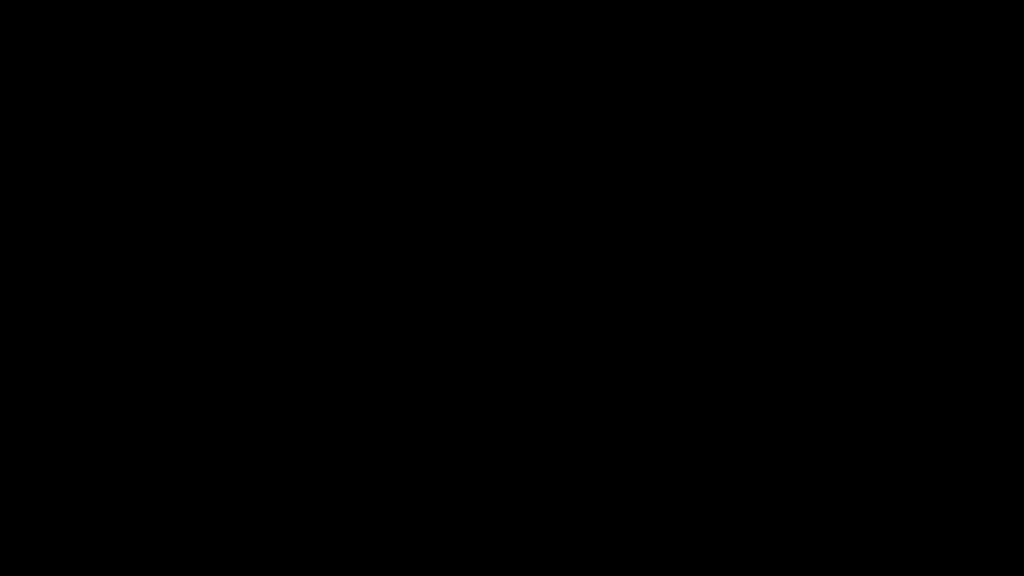
Top Trending AI Girlfriend Companions
Explore the latest and most popular AI girlfriend generators available this month. These innovative tools provide a unique and interactive experience for users seeking companionship through artificial intelligence.
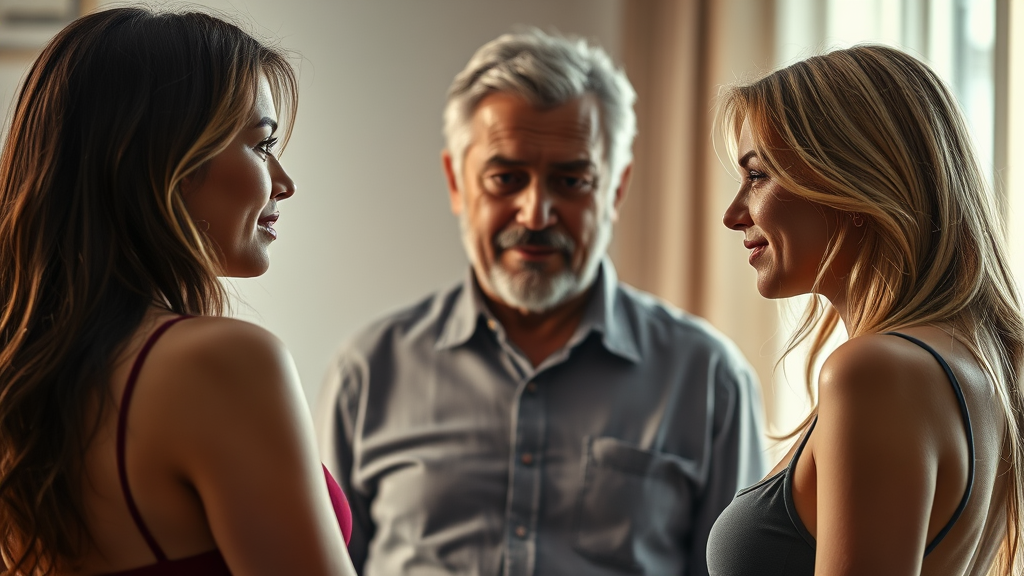
Unlock Exclusive Content with a Free Trial
Experience the unique connection and tailored content that awaits you with our trial offer.
Latest Statistics and Figures
In recent studies, the impact of biases in AI-generated content has come to the forefront, revealing critical issues regarding representation.
- Racial and Gender Biases in AI-Generated Faces:
- Stable Diffusion exhibits significant biases across six races and two genders, depicting nearly all Middle Eastern men as dark-skinned, bearded, and wearing traditional headdresses[1][3][5].
- In datasets generated by Stable Diffusion, White individuals constitute 47% of the images, Black individuals 33%, Asians 3%, and Indians 5%[1].
- Males are overrepresented, making up 65% of all images, while females constitute only 35%[1].
Historical Data for Comparison
- Facial Recognition Bias:
- Historical data shows that facial recognition algorithms have consistently performed better for white faces than for Black faces. For example, a study from 2018 found a correct identification rate of over 99% for white males but only 50% for Black women[2].
Recent Trends or Changes in the Field
- Impact of Inclusive Images:
- Recent studies have shown that exposure to inclusive AI-generated faces can reduce people’s racial and gender biases, while non-inclusive images can increase these biases. This effect is independent of whether the images are labeled as AI-generated[1][3][5].
- There is a growing trend towards developing debiasing solutions, such as fine-tuning models with diverse datasets to achieve more balanced representation in generated images[1].
Relevant Economic Impacts or Financial Data
- Economic and Social Impacts:
- Unaddressed biases in AI systems can hinder people’s ability to participate in the economy and society, reducing the potential benefits of AI. This can lead to mistrust among marginalized groups and distorted results that are economically detrimental[4].
Notable Expert Opinions or Predictions
- Expert Insights on Bias:
- Dr. Alex Hanna from the Distributed AI Research (DAIR) Institute emphasizes that AI bias is not just a problem of the data, but also of who is involved in formulating and framing the problem. Increasing diversity in the development team and the data set is crucial to mitigating bias[2].
- Experts highlight that the construction of AI technologies, such as facial recognition, can inherently be biased due to their disproportionate use in communities of color and other marginalized groups[2].
Key Findings and Solutions
- Racial Homogenization:
- Debiasing Solutions:
- Researchers propose solutions like the SDXL-Inc model, which is fine-tuned using diverse datasets to achieve more balanced representation in generated images. This model has shown significant improvements in reducing racial and gender biases[1].
Frequently Asked Questions
1. What are the main racial and gender biases present in AI-generated faces?
AI-generated faces often reflect and amplify existing racial and gender biases. For example, models like Stable Diffusion misrepresent Middle Eastern men and show significant disparities in face recognition accuracy: over 99% for white males compared to only 50% for Black women.
2. How does representation in AI datasets impact the generated images?
Representation in datasets reveals stark discrimination. For instance, in AI-generated images, White individuals represent 47%, Black individuals 33%, while Asians and Indians account for only 3% and 5%, respectively. Males dominate with 65% representation, and females only comprise 35%.
3. What effects do inclusive and non-inclusive AI-generated images have on viewers?
Exposure to inclusive AI-generated images can significantly reduce racial and gender biases. In contrast, non-inclusive images can enhance these biases, regardless of whether they are labeled as AI-generated. This underscores the importance of diversity in representation.
4. What challenges do AI-generated systems face in creating diverse images?
AI systems face several diversity challenges, especially in portraying mixed-race couples. For example, Meta’s AI consistently struggles to accurately represent Asian and white pairings. Instead, it often defaults to stereotypes, particularly concerning Asian women.
5. How are debiasing solutions improving bias in AI-generated images?
Research initiatives, like the SDXL-Inc model, show that fine-tuning with diverse datasets can lead to improved dataset representation. This method successfully achieved nearly equal representation across all races and genders, contrasting sharply with the performance of previous models such as Stable Diffusion.
6. What key terms should be recognized in discussions about biases in AI imagery?
Key terms include:
- Racial homogenization: Tendency for AI to depict individuals of the same race as similar.
- Bias in training data: Disproportionate representation of certain groups in AI training data.
- Inclusive AI-generated faces: Faces designed to reduce racial and gender biases.
- SDXL-Inc: A model that addresses biases in AI-generated images through improved training.
7. Why is the underrepresentation of certain races and genders a concern in AI?
The underrepresentation of diverse races and genders contributes to dataset disparities, which in turn leads to biased and stereotypical outputs in AI-generated images. This lack of representation can perpetuate harmful stereotypes and fail to reflect societal diversity.
8. What role does exposure play in shaping biases from AI-generated content?
Exposure to inclusive AI-generated faces can significantly influence societal biases. Increased exposure to non-inclusive images, however, can exacerbate pre-existing racial and gender biases, further highlighting the critical need for diversity in models.
9. How can advancements in AI address these biases in the future?
Advancements in AI technology and methodologies are crucial for achieving equitable representation. Ongoing efforts focused on enhancing diversity training and implementing debiasing solutions are steps toward generating more inclusive outcomes in the field of AI-generated imagery.
10. What are some future directions for tackling biases in AI imagery?
Future directions include a continued emphasis on developing debiasing solutions, expanding dataset diversity, and fostering an industry-wide commitment to inclusive outcomes. As technology evolves, so too should our strategies for combating biases within AI.